I wonder if the authors of the Defence Strategic Review released the day before Anzac Day have been paying attention to the sharp acceleration of progress on artificial intelligence that has taken shape over the past decade, and shows every sign of going exponential now?
I mean, really paying attention. They would doubtless claim that, of course, they are cognizant of AI’s military applications, along with other emerging technologies. If so, it is not apparent in the public report that has been released. Here is the sole mention of AI in the report’s 112 pages:
The success of AUKUS is essential for Australia in acquiring asymmetric capability. AUKUS Pillar II Advanced Capabilities will contribute to strengthening the AUKUS partners’ industrial bases, eliminating barriers to information sharing, and technological cooperation. It will develop and deliver advanced capabilities in areas such as artificial intelligence, hypersonics and maritime domain awareness.
That’s it! By contrast, there is a full section on climate change and the clean energy transition, matters that are of some relevance to Australia’s strategic situation, but not in the way the report’s authors seem to have in mind.
I think this shows a disturbing lack of perspective. AI is set to transform just about everything in the years and decades to come, and nothing more than weaponry and defence systems and strategies.
Take, as a particularly pertinent example, AI’s likely impact on anti-submarine warfare. In July last year IEEE Spectrum, the journal of the international Institute of Electrical and Electronic Engineers, published an article titled Will AI Steal Submarine’s Stealth? with the sub-title “Better detection will make the oceans transparent—and perhaps doom mutually assured destruction”.
Among the sources the article draws on is an Australian report released two years earlier (May 2020) prepared by a group of researchers at the National Security College at the ANU with the equally emphatic title Transparent Oceans? The Coming SSBN Counter-Detection Task May be Insuperable.
Both reports refer mainly to ballistic missile submarines, and the prospect that new detection technologies could compromise what was thought to be the most secure element in the American nuclear deterrence triad. However, the same concern would also apply to the nuclear attack submarines Australia is set to acquire through the AUKUS arrangements announced in September 2021.
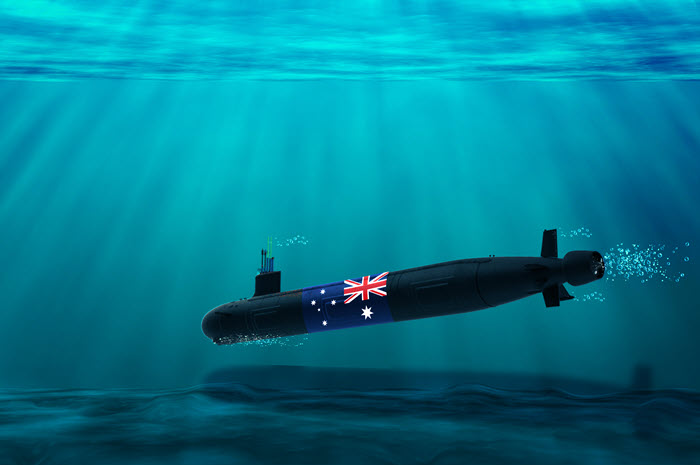
How soon could this happen? The IEEE article refers to the Australian report’s prediction that this could happen as early as 2050. After applying a software tool that is widely used in the intelligence community to provide probabilistic assessments that are “rigorous, transparent and defensible” they conclude the following:
Our assessments showed that the oceans are, in most circumstances, at least likely and, from some perspectives, very likely to become transparent by the 2050s … Even allowing for a generous assumption of progress in counter-detection in our analyses, we cannot see how counter-detection can possibly be as effective in the 2050s as it is today. We are forced to conclude that the coming counter-detection tasks may be insuperable.
Set that against the timeline outlined by the government for Australia’s nuclear submarine acquisition. According to the PM’s statement of March 2023, delivery of three Virginia class subs is to happen “as early as the 2030s”, with the delivery of the new UK-designed AUKUS submarines to start in the 2040s, with completion in the 2050s. The implications seem serious, given the expected service life of these submarines of at least 30 years.
The ANU report focussed primarily on improvements to sensing technologies, the ability to detect anomalies of various kinds created by submarines passing in the deep oceans. In a follow-up article published in March of this year, the authors summarized:
Subs in the ocean are large, metallic anomalies that move in the upper portion of the water column. They produce more than sound. As they pass through the water, they disturb it and change its physical, chemical and biological signatures. They even disturb Earth’s magnetic field – and nuclear subs unavoidably emit radiation. Science is learning to detect all these changes, to the point where the oceans of tomorrow may become ‘transparent’. The submarine era could follow the battleship era and fade into history.
The IEEE Spectrum article, coming two years after the ANU report, added some additional factors such as the ability to detect the tiny surface wake patterns submarines create (Bernoulli Humps), and the importance of persistent satellite coverage of the earth’s oceans.
More importantly, it goes on to describe the role of AI in integrating and interpreting the mass of data produced by sensors of various kinds:
Though these new sensing methods have the potential to make submarines more visible, no one of them can do the job on its own. What might make them work together is the master technology of our time: artificial intelligence … Unlike traditional software, which must be programmed in advance, the machine-learning strategy used here, called deep learning, can find patterns in data without outside help.
The master technology of our time? That is a strong claim, but one that is fully warranted. And what is this “deep learning” that can enable an AI to teach itself?
After a period in the doldrums, sometimes termed the AI winter, progress in artificial intelligence has undergone an extraordinary acceleration in the past decade, with the rate of change steadily accelerating to the point that now important new developments are being announced almost daily. The number of published papers on AI and machine intelligence has grown exponentially since the 1990s.
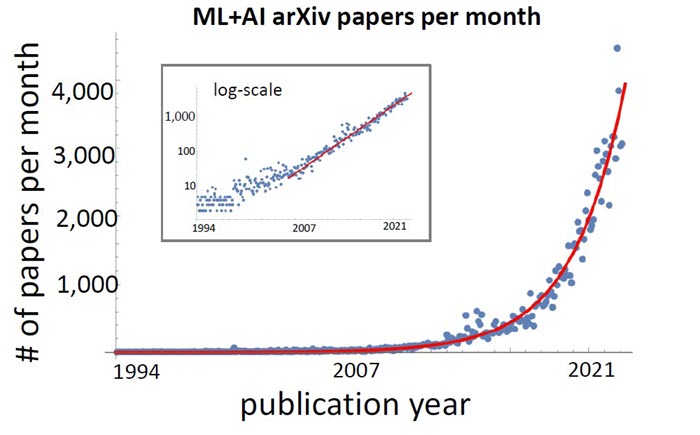
So, what has happened to produce this change? To use the old cliché, the approach to AI development has undergone a paradigm change, shifting from an emphasis on AIs that rely on logic-chopping and symbolic analysis to encode human expertise in some field to an approach that aims to mimic the functioning of the human brain by building large artificial neural networks that can learn for themselves—a shift from “expert systems” to “machine learning”.
The new approach is often termed deep learning, a reference to the multi-layered neural network architectures used in applications like ChatGPT that has caused such as stir since being released in November last year. Much more than just a chatbot, ChatGPT—especially its latest incarnation GPT-4 released in March of this year—has shown an extraordinary range of abilities.
A team of Microsoft researchers, after spending six months evaluating a pre-release version of GPT-4, claimed in a 154-page report that it exhibited “sparks” of artificial general intelligence (AGI), the ability to achieve human or super-human levels of competence in multiple diverse fields. AGI is the Holy Grail of AI research, until recently thought to be several decades away, if it is attainable at all.
If ChatGPT alerted a wider Western public to what was going on in AI, East Asia received its wake-up call back in 2016. That was the year that an AI produced by the British startup DeepMind (acquired by Google in 2014, and now fully integrated into Google’s AI efforts), called AlphaGo, defeated the world champion in the board game Go, popular in Asian countries including Korea, Japan and China.
Achieving this level of play in Go is far more significant than IBM’s chess-playing AI Deep Blue defeating the then world champion, Gary Kasparov, in 1997. Deep Blue exemplified the expert systems approach, encoding as it did a multitude of rules and gameplay strategies drawn from top-level human players. This was combined with sheer computational brute force to search for and evaluate possible moves and strategies to find the best one.
It worked for chess but failed dismally when applied to Go. The rules of Go are superficially straightforward. Two players take turns placing small stones (black and white) on a 19 by 19 lined board, with the aim of encircling the opposing players stones to “capture” territory. The player with the most board territory at the end wins.
The problem is that the number of possible Go board configurations is beyond astronomical, 10 raised to power 170 (10^170 in a common notation). To put that number in perspective, the number of atoms in the observable universe is estimated at around 10^80.
Brute force computation was of no avail when confronted by a combinatorial search explosion of this magnitude. Venerable players believe there is something inherently human, indeed spiritual, about the game, and rely heavily on intuition rather than calculation in choosing moves.
The CEO and founder of DeepMind, Dennis Hassabis, described how AlphaGo was developed in a lecture delivered at Oxford university last year. It used an AI technique called deep reinforcement learning, in which an initially naïve AI gains expertise by playing millions of games against itself, incorporating the gameplay experience to produce successively better versions.
The series of games against the then world champion, South Korean Lee Sedol, received very little publicity in the West, but was a sensation throughout East Asia. There is a full-length documentary (AlphaGo—The Movie) about the tournament on YouTube.
DeepMind followed this up the following year with AlphaZero. Starting with no knowledge whatsoever about the game other than the rules (unlike AlphaGo, which benefited initially from some human expertise), after just eight hours of reinforcement learning through self-play, AlphaZero could beat the best players in the world, including the original AlphaGo. It came up with some winning moves that were completely novel and counter-intuitive to experienced human players.
DeepMind then moved on to applying the same reinforcement learning approach to scientific and technological problems, a major success being a computational solution, AlphaFold, to the protein folding problem, predicting the complex, three-dimensional shape of proteins. This process used to take years using expensive experimental techniques. AlphaFold could work it out in seconds, allowing virtually all 200 million known proteins on the planet to be determined.
According to Kai-Fu Lee, the former president of Google China, the game where AlphaGo beat Lee Sedol was watched by 280 million Chinese. It was a sensation: “to people here, AlphaGo’s victories were both a challenge and an inspiration … they turned into China’s Sputnik moment for artificial intelligence”, leading the regime to announce an ambitious plan to pour resources into AI development with the goal of making China the world leader by 2030.
It was a game with significant implications for the battle for supremacy in AI, the field widely touted as the next great general purpose technological revolution, the outcome of which could well decide whether in future the democracies or autocracies dominate. Vladimir Putin declared several years ago: “whoever becomes the leader in this sphere will become the ruler of the world.”
But back to submarines. For several years the US has been working on a program to apply reinforcement learning to submarine detection, in conjunction with researchers at the Scripps Institute of Oceanography at the University of California San Diego.
Well, that is good to know. But it seems these researchers have some interesting collaborators. Here is some information, from an article on the defence technology site International Defense, Security and Technology:
Scientists from China and the United States have developed a new artificial intelligence-based system that they say will make it easier to detect submarines in uncharted waters … The researchers said the new technology should allow them to track any sound-emitting source—be it a nuclear submarine, a whale or even an emergency beeper from a crashed aircraft—using a simple listening device mounted on a buoy, underwater drone or ship.”
The above article refers to a paper with the arcane title Deep-learning source localization using multi-frequency magnitude-only data that appeared in the July 2019 issue of The Journal of the Acoustical Society of America, which is indeed authored by a combination of American and Chinese authors. It describes an AI technique that allows the location of objects in the deep ocean with significantly greater precision.
So, if I understand this correctly, US researchers at Scripps Oceanography are actually collaborating with colleagues from their countries’ chief strategic rival on the development of a sophisticated new technique with clear application to anti-submarine warfare.
Reading this, you wonder about the limits of human naivete. This looks like another instance of a disturbing inclination of some Western universities and other institutions to give away the intellectual farm to a regime bent on becoming the global hegemon of a new autocratic world order. It may have dual uses, but under the CCP regime’s doctrine of military-civil fusion, all research results must be made available on demand to the military.
A recurring feature of AI developments in the past decade is the contraction of time frames to the achievement of key benchmarks. Winning at Go, for example, was thought to be at least a decade away. Artificial General Intelligence was seen as several decades away if it is achievable at all.
Now, some of the most experienced researchers in the field speculate that it could come much earlier, like the Microsoft researchers who think GPT-4 shows “sparks” of AGI. Such has been the speed of these developments that 1300 people working in the field recently signed an open letter calling for a six month pause to assess the “profound risks to society and humanity” these systems could pose.
The critics were joined by the man often referred to as the Godfather of AI, the British scientist Geoffrey Hinton, who back in the 1980s laid the foundations for the deep learning revolution now unfolding. He set out his concerns in a long interview, just published in the New York Times:
The idea that this stuff could actually get smarter than people — a few people believed that … I thought it was 30 to 50 years or even longer away. Obviously, I no longer think that.”
Given which, the spectre of transparent oceans could eventuate much earlier than the 2050s timeframe predicted by the ANU researchers. As with the protein folding problem mentioned above, a powerful AI-enabled solution could emerge quite suddenly.
Hopefully, Australia’s defence planners are devoting a great deal of thought to how the future submarines will be deployed in this eventuality, almost certainly in conjunction with fleets of autonomous sub-surface drones, a rapidly emerging technology with some Australian participation.
But Australia’s capabilities are limited. Close engagement with democratic allies though the network of alliances and associations that have emerged in recent years (AUKUS, the Quad, closer liaison with NATO) will be essential to secure Australia’s safety during this dangerous time.
Given the speed and uncertainty of AI-driven technological change, it is crucial that decision makers keep a flexible mindset capable of adjusting to emerging developments. The last thing needed is a plodding bureaucratic “plough on regardless” mentality at a time of one of the most rapid and epochal technological transformations in human history.